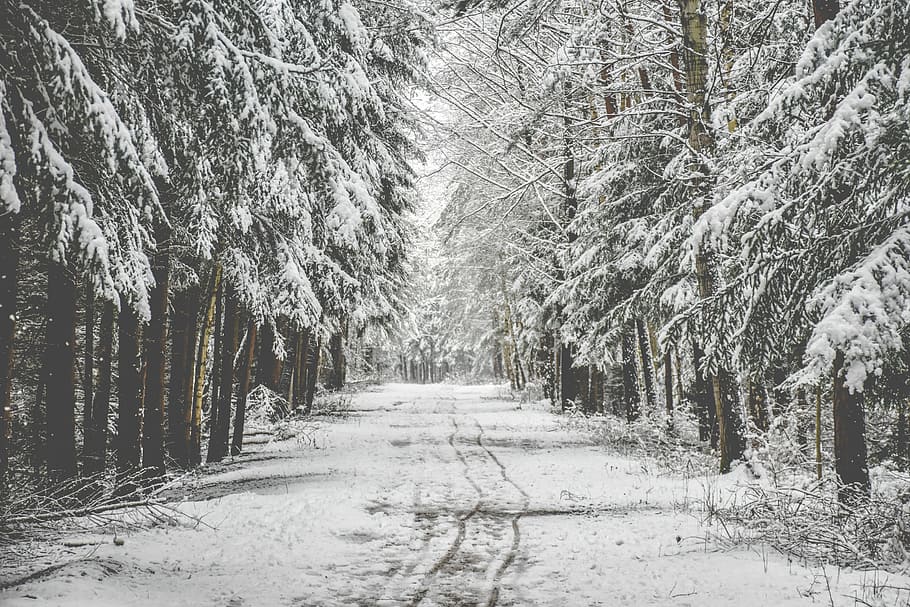
ESSIC/CISESS scientist Soni Yatheendradas is a co-author on a paper in Journal of Hydrometeorology titled, “A novel Machine Learning-based gap-filling of fine-resolution remotely sensed snow cover fraction data by combining downscaling and regression”.
Satellite-based remotely-sensed observations of snow cover fraction (SCF) can have data gaps in spatially distributed coverage from sensor and orbital limitations. In this study, the researchers worked to mitigate these limitations in the example fine-resolution Moderate Resolution Imaging Spectroradiometer (MODIS) data. Yatheenraas and Kumar used auxiliary 1-km datasets that either aid in downscaling from coarser-resolution (5 km) MODIS SCF wherever not fully covered by clouds, or else by themselves via regression wherever fully cloud-covered. The study’s prototype predicts a 1-km version of the 500 m MOD10A1 SCF target. The generalized partial convolution developed in the study is fully gap-agnostic and can enable multiple earth science applications like downscaling, regression, classification and segmentation that were hindered by data gaps.
Yatheendradas is affiliated with the Hydrological Sciences Lab at NASA GSFC. His current work include leading optimization/uncertainty components on NASA’s Land Information System (LIS) software especially for SUSMAP applications, Machine and Deep Learning applied to spatial downscaling and retrieval in snow remote sensing, and multivariate hydrologic data assimilation for model structural learning and process-diagnostics.
To access the article, click here: “A novel Machine Learning-based gap-filling of fine-resolution remotely sensed snow cover fraction data by combining downscaling and regression”.