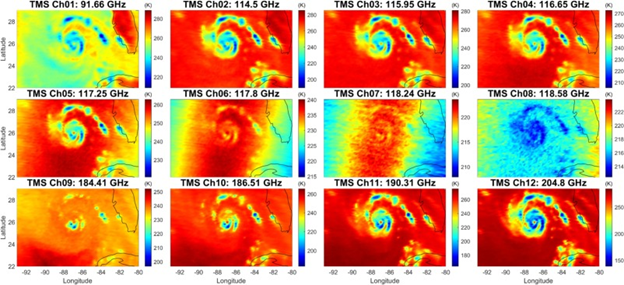
Evaluating Rainfall and Graupel Retrieval Performance of the NASA TROPICS Pathfinder Through the NOAA MiRS System
ESSIC scientists involved with the NASA TROPICS CubeSat mission recently built an algorithm for hydrometers retrieval. In a paper in Remote Sensing of Environment, John