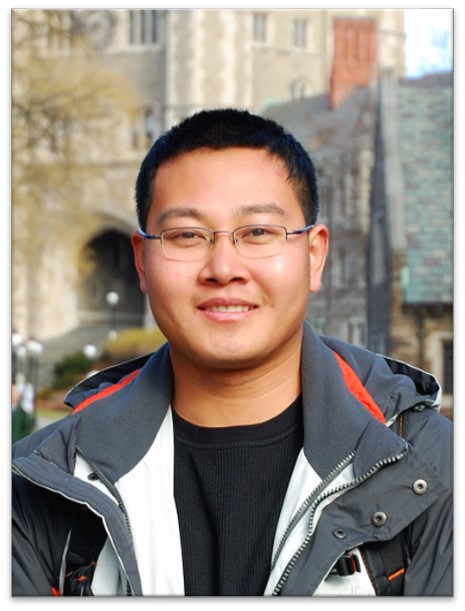
Enhancement of NOAA Snowfall Product Through Machine Learning
This event has passed. See the seminar recording here:
Dr. Yongzhen Fan
Earth System Science Interdisciplinary Center
University of Maryland
Monday November 22, 2021, 2 PM ET
Abstract:
Snowfall accounts for a large fraction of winter precipitation in mid- and high- latitudes. It is also an important indicator of climate change. The NOAA NESDIS operational Snowfall Rate (SFR) product is generated at near real time from a suite of PMW sensors onboard several polar orbiting satellites operated by NOAA and its partner agencies. It estimates liquid equivalent snowfall rate over global land and has been in operation since 2012. The SFR algorithm consists of two main components: snowfall detection (SD) and snowfall rate estimation. The SD algorithm utilizes a logistic regression model which performs well in relatively warm regions but shows inferior performance or is inapplicable under colder weather conditions. The reduced accuracy and lack of retrievals in colder weather are major obstacles to applications in areas where snowfall observations are scarcest such as the western US and most of Alaska. We recently developed machine learning (ML) algorithms to extend SD to extremely cold conditions and to improve its overall performance. We built a global precipitation dataset that consists of collocated ground weather reports, satellite measurements, and NWP model analysis. SD models were developed using several ML techniques and validated globally. I will present the development of the ML SD algorithms for ATMS and MHS sensors and their global validation results. The presentation will also include some ML-based enhancement to the snowfall rate estimation.
Biosketch:
Yongzhen Fan is an assistant research scientist with the Earth System Science Interdisciplinary Center (ESSIC) and the Cooperative Institute for Satellite Earth System Studies (CISESS)-Maryland, University of Maryland (UMD), College Park. He received his B.E. in Electronic Science from Xi’an University of Science and Technology, China and his M.E. in Electrical Engineering and Ph.D. in Physics from Stevens Institute of Technology. His research interests include radiative transfer modeling of the atmosphere, aerosols, cloud and ocean, machine learning, and inverse method. Before joining ESSIC/UMD, he was a postdoctoral researcher at Stevens Institute of Technology and developed a machine learning based data analysis platform for satellite ocean color sensors, Ocean Color – Simultaneous Marine and Aerosol Retrieval Tool (OC-SMART). He was also a research scientist at the Geminor Inc. and developed a polarized coupled atmosphere/surface radiative transfer model, AccuRT. He joined the ESSIC/UMD in 2020 to work on the enhancement of the NOAA Snowfall products through machine learning, improvement of the radiative transfer simulation, and ice cloud microphysics.
Webinar:
Webinar: https://go.umd.edu/fanwebinar
Event site: https://go.umd.edu/fan
Webinar number: 2624 189 8397
Webinar password: essic
To join the audio conference only:
US Toll: +1-415-655-0002
Global call-in numbers
For IT assistance:
Cazzy Medley: cazzy@umd.edu
Resources:
Seminar schedule & archive: https://go.umd.edu/essicseminar
Seminar Google calendar: https://go.umd.edu/essicseminarcalendar
Seminar recordings on Youtube: https://www.youtube.com/user/ESSICUMD